Narrowing the confidence gap for wider AI adoption
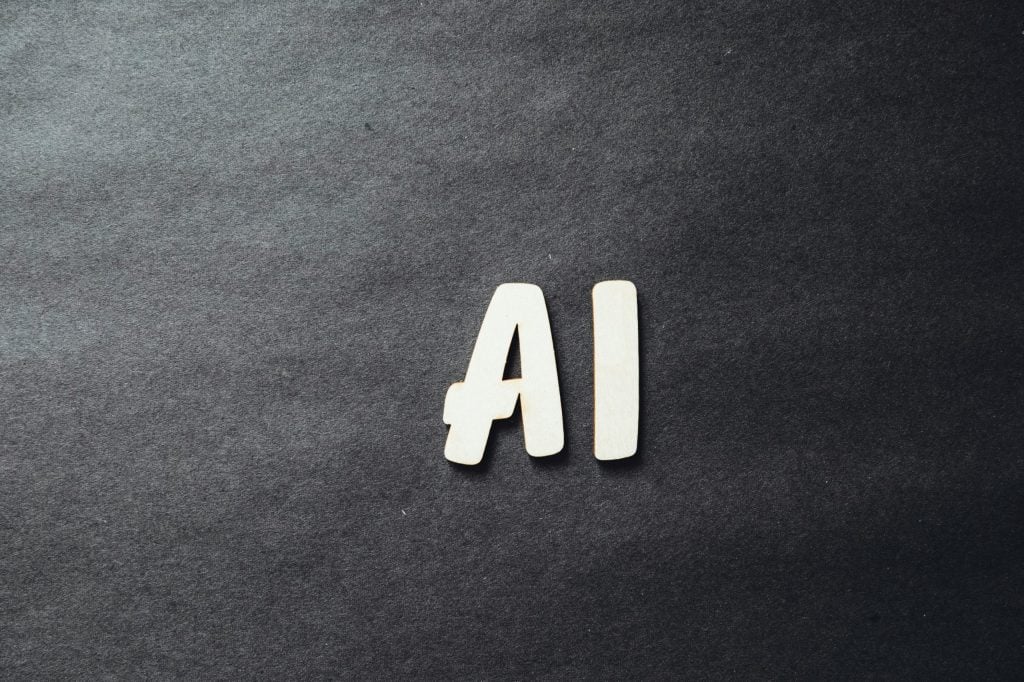
Artificial intelligence entered the market with a splash, driving massive buzz and adoption. But now the pace is faltering.
Business leaders still talk the talk about embracing AI, because they want the benefits – McKinsey estimates that GenAI could save companies up to $2.6 trillion across a range of operations. However, they aren’t walking the walk. According to one survey of senior analytics and IT leaders, only 20% of GenAI applications are currently in production.
Why the wide gap between interest and reality?
The answer is multifaceted. Concerns around security and data privacy, compliance risks, and data management are high-profile, but there’s also anxiety about AI’s lack of transparency and worries about ROI, costs, and skill gaps. In this article, we’ll examine the barriers to AI adoption, and share some measures that business leaders can take to overcome them.
Get a handle on data
“High-quality data is the cornerstone of accurate and reliable AI models, which in turn drive better decision-making and outcomes,” said Rob Johnson, VP and Global Head of Solutions Engineering at SolarWinds, adding, “Trustworthy data builds confidence in AI among IT professionals, accelerating the broader adoption and integration of AI technologies.”
Today, only 43% of IT professionals say they’re confident about their ability to meet AI’s data demands. Given that data is so vital for AI success, it’s not surprising that data challenges are an oft-cited factor in slow AI adoption.
The best way to overcome this hurdle is to go back to data basics. Organisations need to build a strong data governance strategy from the ground up, with rigorous controls that enforce data quality and integrity.
Take ethics and governance seriously
With regulations mushrooming, compliance is already a headache for many organisations. AI only adds new areas of risk, more regulations, and increased ethical governance issues for business leaders to worry about, to the extent that security and compliance risk was the most-cited concern in Cloudera’s State of Enterprise AI and Modern Data Architecture report.
While the rise in AI regulations might seem alarming at first, executives should embrace the support that these frameworks offer, as they can give organisations a structure around which to build their own risk controls and ethical guardrails.
Developing compliance policies, appointing teams for AI governance, and ensuring that humans retain authority over AI-powered decisions are all important steps in creating a comprehensive system of AI ethics and governance.
Reinforce control over security and privacy
Security and data privacy concerns loom large for every business, and with good reason. Cisco’s 2024 Data Privacy Benchmark Study revealed that 48% of employees admit to entering non-public company information into GenAI tools (and an unknown number have done so and won’t admit it), leading 27% of organisations to ban the use of such tools.
The best way to reduce the risks is to limit access to sensitive data. This involves doubling down on access controls and privilege creep, and keeping data away from publicly-hosted LLMs. Avi Perez, CTO of Pyramid Analytics, explained that his business intelligence software’s AI infrastructure was deliberately built to keep data away from the LLM, sharing only metadata that describes the problem and interfacing with the LLM as the best way for locally-hosted engines to run analysis.”There’s a huge set of issues there. It’s not just about privacy, it’s also about misleading results. So in that framework, data privacy and the issues associated with it are tremendous, in my opinion. They’re a showstopper,” Perez said. With Pyramid’s setup, however, “the LLM generates the recipe, but it does it without ever getting [its] hands on the data, and without doing mathematical operations. […] That eliminates something like 95% of the problem, in terms of data privacy risks.”
Boost transparency and explainability
Another serious obstacle to AI adoption is a lack of trust in its results. The infamous story of Amazon’s AI-powered hiring tool which discriminated against women has become a cautionary tale that scares many people away from AI. The best way to combat this fear is to increase explainability and transparency.
“AI transparency is about clearly explaining the reasoning behind the output, making the decision-making process accessible and comprehensible,” said Adnan Masood, chief AI architect at UST and a Microsoft regional director. “At the end of the day, it’s about eliminating the black box mystery of AI and providing insight into the how and why of AI decision-making.”Unfortunately, many executives overlook the importance of transparency. A recent IBM study reported that only 45% of CEOs say they are delivering on capabilities for openness. AI champions need to prioritise the development of rigorous AI governance policies that prevent black boxes arising, and invest in explainability tools like SHapley Additive exPlanations (SHAPs), fairness toolkits like Google’s Fairness Indicators, and automated compliance checks like the Institute of Internal Auditors’ AI Auditing Framework.
Define clear business value
Cost is on the list of AI barriers, as always. The Cloudera survey found that 26% of respondents said AI tools are too expensive, and Gartner included “unclear business value” as a factor in the failure of AI projects. Yet the same Gartner report noted that GenAI had delivered an average revenue increase and cost savings of over 15% among its users, proof that AI can drive financial lift if implemented correctly.
This is why it’s crucial to approach AI like every other business project – identify areas that will deliver fast ROI, define the benefits you expect to see, and set specific KPIs so you can prove value.”While there’s a lot that goes into building out an AI strategy and roadmap, a critical first step is to identify the most valuable and transformative AI use cases on which to focus,” said Michael Robinson, Director of Product Marketing at UiPath.
Set up effective training programs
The skills gap remains a significant roadblock to AI adoption, but it seems that little effort is being made to address the issue. A report from Worklife indicates the initial boom in AI adoption came from early adopters. Now, it’s down to the laggards, who are inherently sceptical and generally less confident about AI – and any new tech.
This makes training crucial. Yet according to Asana’s State of AI at Work study, 82% of participants said their organisations haven’t provided training on using generative AI. There’s no indication that training isn’t working; rather that it isn’t happening as it should.
The clear takeaway is to offer comprehensive training in quality prompting and other relevant skills. Encouragingly, the same research shows that even using AI without training increases people’s skills and confidence. So, it’s a good idea to get started with low- and no-code tools that allow employees who are unskilled in AI to learn on the job.
The barriers to AI adoption are not insurmountable
Although AI adoption has slowed, there’s no indication that it’s in danger in the long term. The many obstacles holding companies back from rolling out AI tools can be overcome without too much trouble. Many of the steps, like reinforcing data quality and ethical governance, should be taken regardless of whether or not AI is under consideration, while other steps taken will pay for themselves in increased revenue and the productivity gains that AI can bring.