Capgemini digs into the real reasons that gen AI proof of concepts rarely take off
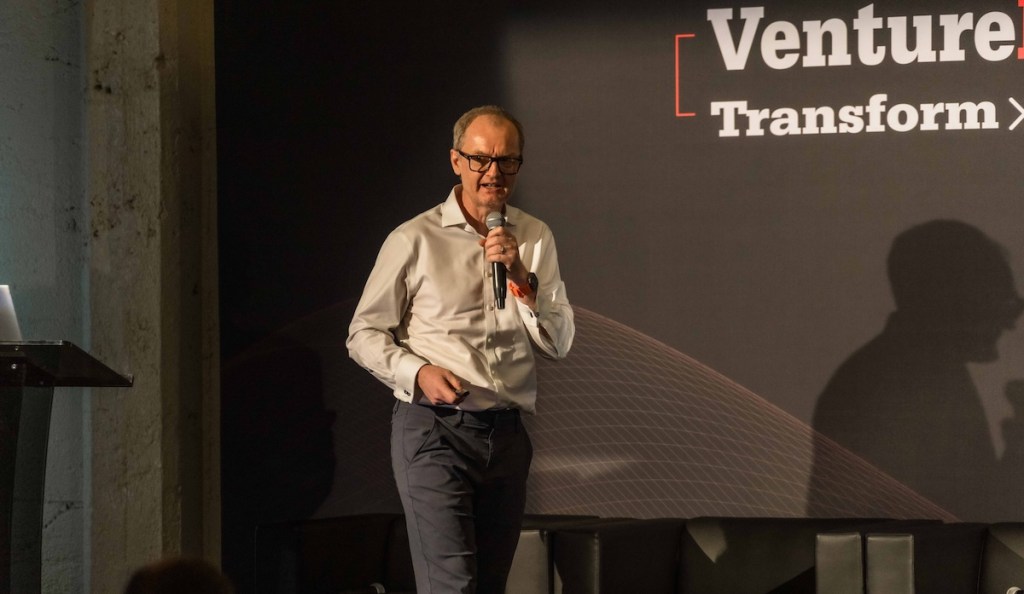
According to recent Capegemini research, the vast majority of people in every sector they’ve surveyed have said they have a massive problem turning AI proof of concepts into production solutions. And the reason behind the lag is digital boundaries, digital employees and bad data, Steve Jones, EVP, data driven business and gen AI at Capgemini explained to attendees at VB Transform.
“We have become very comfortable in a world of bad data, and I speak as a data guy,” Jones said. “We have been very comfortable with the biggest myth in everybody’s IT status being that we will fix it in the source system — it’s the biggest lie that any organization tells themselves about data, historically.”
He went on to explain that a big chunk of the reason that data is often referred to as the new oil is because oil’s only useful after refinement. In a world where 50% of business decisions will be made by AI by 2030 — that’s to say, primarily in autonomous supply chain applications — that’s unacceptable from a risk perspective. And it poses a profound risk from a data perspective.
“If I have a digital employee that’s making a decision, they cannot be waiting for cleaned up data because that’s not going to work operationally,” he added. “If you are working in an autonomous vehicle, it is no good. If you’re working in an autonomous warehouse, it’s no good. We should be thinking about how we will have digital employees in organizations. How it will be the business responsibility and the business success to be able to manage not just the people in their team, but to be able to manage the AI in the team.”
LLMs are will do phenomenally stupid things unless they have access to information that represents the operational reality of the business. Unfortunately, he says, businesses have spent 50 years building up a separation between the operational side of the business and the data side of the business.
So how does the AI adoption issue get solved?
A critical need for digital boundaries
The first step is to develop a digital operating model. In other words: Can you digitally describe the problem you’re trying to solve? Do you have a boundary description that outlines not just what the problem is to solve, but what it should not do? For example, when you look at data, can you say which data should be used to drive a decision, and which data should not be used to drive a decision? What should AI be allowed to influence, what should it not be allowed to influence? And can you describe all of that in a way that an AI can process and be bound by?
“If you create a phenomenal AI whose job it is to reduce the carbon impact of a business and you roll it out to an oil company, the greatest way within an oil company to reduce the carbon impact of the oil company is to stop being an oil company,” he said. “That isn’t a very successful business strategy. Therefore, you have to think, how have I digitally ensured that it is doing what I want it to do within the boundaries of what my business is.”
Moving forward, no organization is going to end up with an AI brain that manages everything in the company — in large part because from a risk management and cyber threat perspective alone, that’s far too high a level of risk. More importantly, that isn’t how a business works, and that isn’t how a business will adopt it, nor is it how a business can manage it.
Every AI solution in a company will be constrained by its function. For example, the debt collection bot responsible to the finance department will be constrained by a very different set of rules, regulations and motivations than the sales advisor bot — and that’s how business works, in functions and departments. And part of the reason that so many organizations are having such a hard time moving from proof of concept to wholesale AI adoption is that companies are not considering AI through a business adoption and management lens, and instead continue to hold out for the AI technology that will solve all of its problems.
“We’re thinking about technology and the idea that this will solve everything — that won’t help a business adopt it because people cannot adopt it,” he added. “When I look at modeling these business problems, I’m modeling them in the smallest level of granularity that enables me to bound it from a cyber risk perspective, from a business risk perspective, and to be able to define that contract.”
For instance, a sales advisor bot is working and collaborating with four sub-robots. Those sub-robots each have their own bounds and contract, each have their own things they can and cannot do, and it is the collaboration of those which is driving the business outcome. We need to start thinking about AI at this level because the next stage, and the next challenge, is that these digital employees are going to have to collaborate with people and with each other. They’re going to have to ask questions, and they’ll be asking both people and other agents within digital employees within the organization. Without very clear boundaries, the risk is huge and the cyber threat enormous.
“However, if each one of these is bounded, if each one of these is controlled, if each one of these is accountable to the area of the business, I can then start doing automations that I’ve fundamentally never been able to do,” Jones said. “I can start doing business processes and shifting the abstraction to a level that I’d never been able to do, but I’m only going to do that if I approach it from the perspective of automating and looking at the business model, not looking at a series of steps and trying to put a little bit of AI in each one of the steps.”
Organizational change to scale AI
“We need to think about the organizational change to scale this up, not the technology change,” Jones said. “The technology change? We’re in Silicon Valley. This is where technology change, I would say, safely is not a problem. The problem of adoption is a business adoption problem, is a business model problem. We have to think about data architecture for AI as being fundamentally different.”
That means application design needs to change. Where historically in application design the data lives in the back end where transactions take place, transactions are the least important thing for AI in an application. Data needs to be up front where the digital employees are using data in the moment to complete tasks accurately and effectively.
The reason why the movement from proof of concept to full scale AI adoption is so low is that the current data approach is not the destination we need, he added.
“Digital employees will require us to be in control of our digital operating model and most organizations today fundamentally are not,” he explained. “To understand the business context will be central to being able to deploy those digital employees. That means that the organization will change more than the technology. We are asking business people who do not understand technology to delegate their career to their engagement with AI. That’s the challenge that we are tasked with. To do that, to move to a world in which the 50% AI world exists, it means we need to enable business people to be successful in their careers by relying on AI.”