LLM progress is slowing — what will it mean for AI?
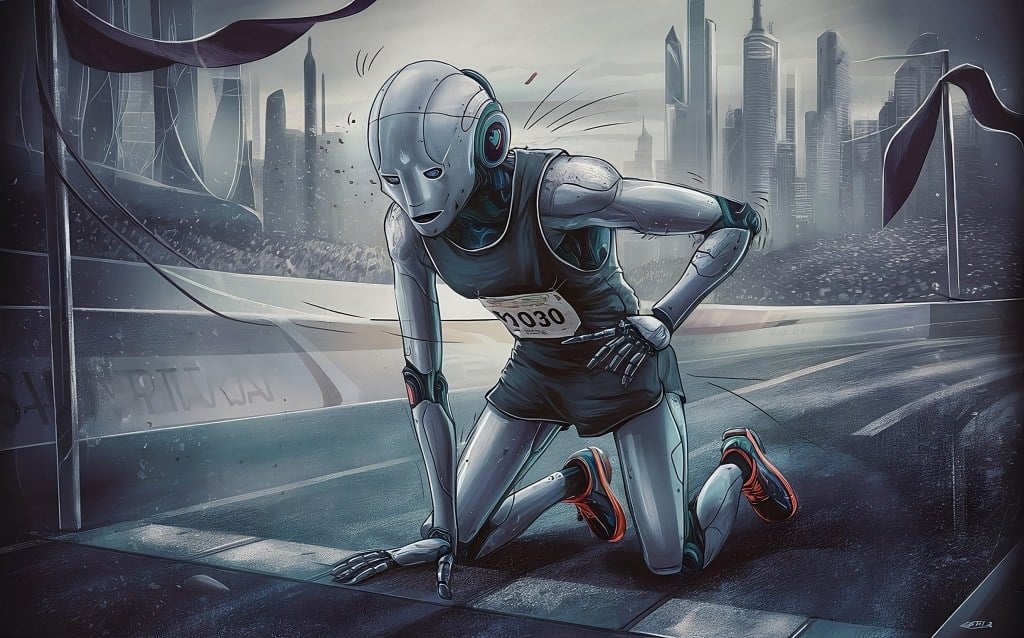
Join our daily and weekly newsletters for the latest updates and exclusive content on industry-leading AI coverage. Learn More
We used to speculate on when we would see software that could consistently pass the Turing test. Now, we have come to take for granted not only that this incredible technology exists — but that it will keep getting better and more capable quickly.
It’s easy to forget how much has happened since ChatGPT was released on November 30, 2022. Ever since then, the innovation and power just kept coming from the public large language models LLMs. Every few weeks, it seemed, we would see something new that pushed out the limits.
Now, for the first time, there are signs that that pace might be slowing in a significant way.
To see the trend, consider OpenAI’s releases. The leap from GPT-3 to GPT-3.5 was huge, propelling OpenAI into the public consciousness. The jump up to GPT-4 was also impressive, a giant step forward in power and capacity. Then came GPT-4 Turbo, which added some speed, then GPT-4 Vision, which really just unlocked GPT-4’s existing image recognition capabilities. And just a few weeks back, we saw the release of GPT-4o, which offered enhanced multi-modality but relatively little in terms of additional power.
Other LLMs, like Claude 3 from Anthropic and Gemini Ultra from Google, have followed a similar trend and now seem to be converging around similar speed and power benchmarks to GPT-4. We aren’t yet in plateau territory — but do seem to be entering into a slowdown. The pattern that is emerging: Less progress in power and range with each generation.
This will shape the future of solution innovation
This matters a lot! Imagine you had a single-use crystal ball: It will tell you anything, but you can only ask it one question. If you were trying to get a read on what’s coming in AI, that question might well be: How quickly will LLMs continue to rise in power and capability?
Because as the LLMs go, so goes the broader world of AI. Each substantial improvement in LLM power has made a big difference to what teams can build and, even more critically, get to work reliably.
Think about chatbot effectiveness. With the original GPT-3, responses to user prompts could be hit-or-miss. Then we had GPT-3.5, which made it much easier to build a convincing chatbot and offered better, but still uneven, responses. It wasn’t until GPT-4 that we saw consistently on-target outputs from an LLM that actually followed directions and showed some level of reasoning.
We expect to see GPT-5 soon, but OpenAI seems to be managing expectations carefully. Will that release surprise us by taking a big leap forward, causing another surge in AI innovation? If not, and we continue to see diminishing progress in other public LLM models as well, I anticipate profound implications for the larger AI space.
Here is how that might play out:
More specialization: When existing LLMs are simply not powerful enough to handle nuanced queries across topics and functional areas, the most obvious response for developers is specialization. We may see more AI agents developed that take on relatively narrow use cases and serve very specific user communities. In fact, OpenAI launching GPTs could be read as a recognition that having one system that can read and react to everything is not realistic.
Rise of new UIs: The dominant user interface (UI) so far in AI has unquestionably been the chatbot. Will it remain so? Because while chatbots have some clear advantages, their apparent openness (the user can type any prompt in) can actually lead to a disappointing user experience. We may well see more formats where AI is at play but where there are more guardrails and restrictions guiding the user. Think of an AI system that scans a document and offers the user a few possible suggestions, for example.
Open source LLMs close the gap: Because developing LLMs is seen as incredibly costly, it would seem that Mistral and Llama and other open source providers that lack a clear commercial business model would be at a big disadvantage. That might not matter as much if OpenAI and Google are no longer producing huge advances, however. When competition shifts to features, ease of use, and multi-modal capabilities, they may be able to hold their own.
The race for data intensifies: One possible reason why we’re seeing LLMs starting to fall into the same capability range could be that they are running out of training data. As we approach the end of public text-based data, the LLM companies will need to look for other sources. This may be why OpenAI is focusing so much on Sora. Tapping images and video for training would mean not only a potential stark improvement in how models handle non-text inputs, but also more nuance and subtlety in understanding queries.
Emergence of new LLM architectures: So far, all the major systems use transformer architectures but there are others that have shown promise. They were never really fully explored or invested in, however, because of the rapid advances coming from the transformer LLMs. If those begin to slow down, we could see more energy and interest in Mamba and other non-transformer models.
Final thoughts: The future of LLMs
Of course, this is speculative. No one knows where LLM capability or AI innovation will progress next. What is clear, however, is that the two are closely related. And that means that every developer, designer and architect working in AI needs to be thinking about the future of these models.
One possible pattern that could emerge for LLMs: That they increasingly compete at the feature and ease-of-use levels. Over time, we could see some level of commoditization set in, similar to what we’ve seen elsewhere in the technology world. Think of, say, databases and cloud service providers. While there are substantial differences between the various options in the market, and some developers will have clear preferences, most would consider them broadly interchangeable. There is no clear and absolute “winner” in terms of which is the most powerful and capable.
Cai GoGwilt is the co-founder and chief architect of Ironclad.
DataDecisionMakers
Welcome to the VentureBeat community!
DataDecisionMakers is where experts, including the technical people doing data work, can share data-related insights and innovation.
If you want to read about cutting-edge ideas and up-to-date information, best practices, and the future of data and data tech, join us at DataDecisionMakers.
You might even consider contributing an article of your own!
Read More From DataDecisionMakers