Researchers from Stanford and Amazon Developed STARK: A Large-Scale Semi-Structure Retrieval AI Benchmark on Textual and Relational Knowledge Bases
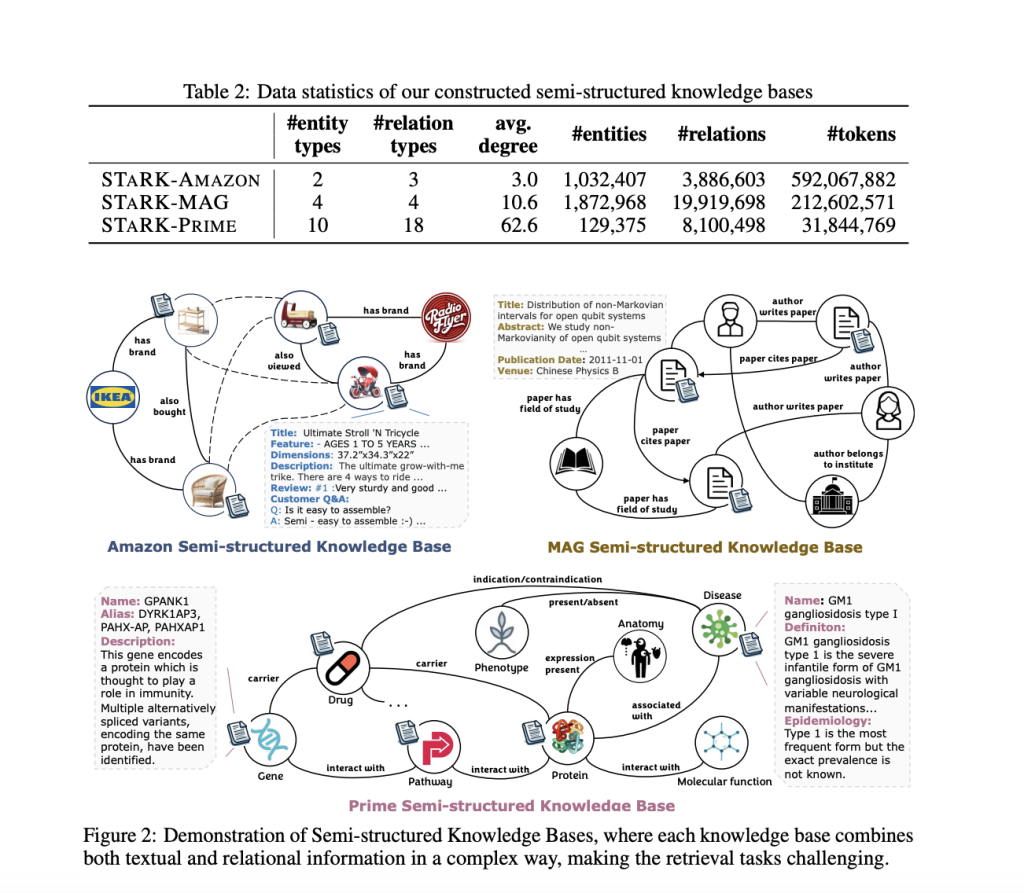
Imagine you’re looking for the perfect gift for your kid – a fun yet safe tricycle that ticks all the boxes. You might search with a query like “Can you help me find a push-along tricycle from Radio Flyer that’s both fun and safe for my kid?” Sounds pretty specific, right? But what if the search engine could understand the textual requirements (“fun” and “safe for kids”) as well as the relational aspect (“from Radio Flyer”)?
This is the kind of complex, multimodal retrieval challenge that researchers aimed to tackle with STARK (Semi-structured Retrieval on Textual and Relational Knowledge Bases). While we have benchmarks for retrieving information from either pure text or structured databases, real-world knowledge bases often blend these two elements. Think e-commerce platforms, social media, or biomedical databases—they all contain a mix of textual descriptions and connections between entities.
To create the benchmark, they first built three semi-structured knowledge bases from public datasets: one about Amazon products, one about academic papers and authors, and one about biomedical entities like diseases, drugs, and genes. These knowledge bases contained millions of entities and relationships between them, as well as textual descriptions for many entities.
Next, they developed a novel pipeline (shown in Figure 3) to automatically generate queries for their benchmark datasets. The pipeline starts by sampling a relational requirement, like “belongs to the brand Radio Flyer” for products. It then extracts relevant textual properties from an entity that satisfies this requirement, such as describing a tricycle as “fun and safe for kids.” Using language models, it combines the relational and textual information into a natural-sounding query, like “Can you help me find a push-along tricycle from Radio Flyer that’s both fun and safe for my kid?”
The really cool part is how they construct the ground truth answers for each query. They take the remaining candidate entities (excluding the one used to extract textual properties) and verify if they actually meet the full query requirements using multiple language models. Only the entities that pass this stringent verification get included in the final ground truth answer set.
After generating thousands of such queries across the three knowledge bases, the researchers analyzed the data distribution and had people evaluate the naturalness, diversity, and practicality of the queries. The results showed that their benchmark captured a wide range of query styles and real-world scenarios.
When they tested various retrieval models on the STARK benchmark, they found that current approaches still struggle with accurately retrieving relevant entities, especially when the queries involve reasoning over both textual and relational information. The best results came from combining traditional vector similarity methods with language model rerankers like GPT-4, but even then, the performance left significant room for improvement. Traditional embedding methods lacked the advanced reasoning capabilities of large language models, while fine-tuning LLMs for this task proved computationally demanding and difficult to align with textual requirements. On the biomedical dataset, STARK-PRIME, the best method could only retrieve the top-ranked correct answer around 18% of the time (as measured by the Hit@1 metric). The Recall@20 metric, which looks at the proportion of relevant items in the top 20 results, remained below 60% across all datasets.
The researchers emphasize that STARK sets a new benchmark for evaluating retrieval systems on SKBs, offering valuable opportunities for future research. They suggest that reducing retrieval latency and incorporating strong reasoning abilities into the retrieval process are prospective directions for advancements in this domain. Additionally, they have made their work open-source, fostering further exploration and development in multimodal retrieval tasks.
Check out the Paper. All credit for this research goes to the researchers of this project. Also, don’t forget to follow us on Twitter. Join our Telegram Channel, Discord Channel, and LinkedIn Group.
If you like our work, you will love our newsletter..
Don’t Forget to join our 40k+ ML SubReddit
Vineet Kumar is a consulting intern at MarktechPost. He is currently pursuing his BS from the Indian Institute of Technology(IIT), Kanpur. He is a Machine Learning enthusiast. He is passionate about research and the latest advancements in Deep Learning, Computer Vision, and related fields.