This AI Paper Introduces the Kolmogorov-Test: A Compression-as-Intelligence Benchmark for Evaluating Code-Generating Language Models
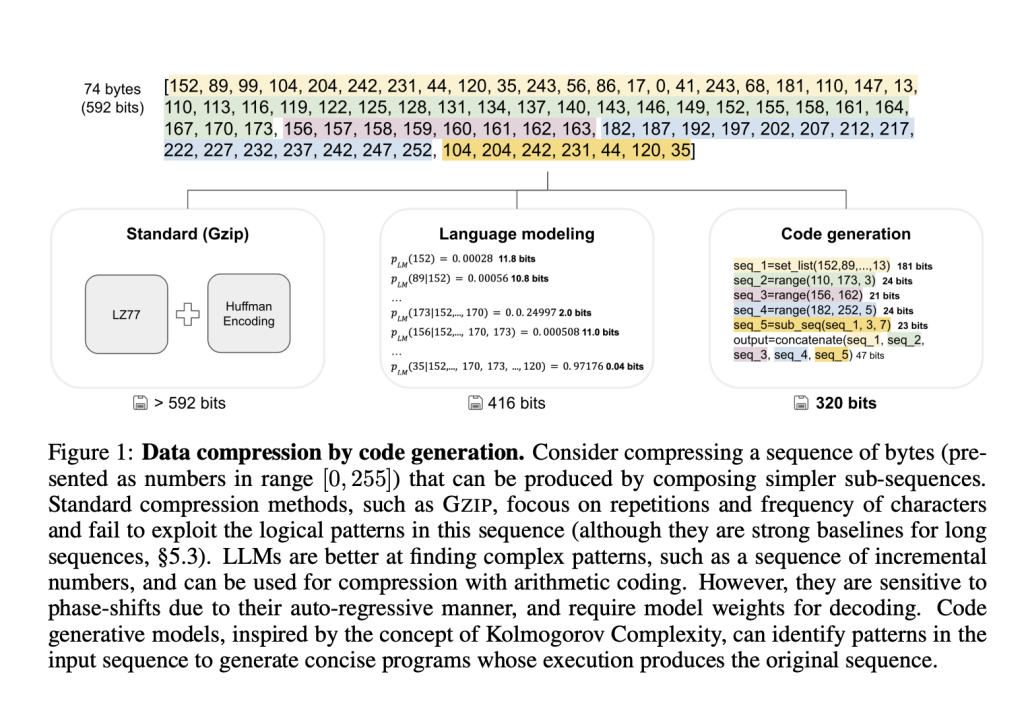
Compression is a cornerstone of computational intelligence, deeply rooted in the theory of Kolmogorov complexity, which defines the minimal program needed to reproduce a given sequence. Unlike traditional compression methods that look for repetition and redundancy, Kolmogorov’s framework interprets compression as a problem of discovering structured patterns through programmatic representation. While the theory promises optimal compression, its uncomputability poses a significant hurdle. Nevertheless, the emergence of large language models capable of code generation opens an intriguing opportunity to test how closely modern systems can approximate this theoretical ideal by reasoning through code rather than pattern matching.
A core issue arises from the limitations of current tools in compressing data sequences using concise, executable code. Models often replicate inputs rather than generate programs that reproduce them, indicating a gap in true pattern understanding. This becomes especially evident when dealing with real-world audio, text, or DNA sequences, where complex logical structures must be uncovered to achieve efficient compression. The main challenge is ensuring the model replicates the sequence and uses a minimal and rational set of instructions. Furthermore, though synthetic training data is useful for controlled evaluation, it often fails to support robust generalization to natural data, which is essential for practical applications.
Several compression tools exist, ranging from traditional algorithms like GZIP to newer neural compression systems. GZIP remains a strong baseline, especially for long or repetitive sequences, due to its effective encoding of statistical regularities. More recently, language modeling approaches have integrated with arithmetic coding, using prediction probabilities to compress input data. However, these methods typically require access to the full model weights at decoding time, limiting their efficiency and applicability. Prompted code-generating models like GPT-4 and LLaMA have also been evaluated in zero-shot settings to generate Python programs that reproduce input sequences. Yet, they frequently produce lengthy, imprecise code with limited success, particularly when faced with unseen or complex sequences.
Researchers from Meta AI and Tel Aviv University introduced the Kolmogorov-Test (KT), a benchmark for assessing the reasoning capability of code-generating language models. The test evaluates a model’s ability to generate the shortest program that outputs a given input sequence. Unlike typical benchmarks, KT emphasizes logical composition and program generation over predictive text modeling. Sequences include natural data from audio (LibriSpeech), text (Wikipedia enwik9), and DNA (GRCh38), as well as synthetic sequences generated through a custom-designed domain-specific language (DSL). This DSL supports building structured sequences by composing operations like range creation, sequence modification, merging, and filtering.
The researchers developed an automated framework to generate millions of synthetic program-sequence pairs using this DSL. These programs then train and evaluate models, including large pre-trained and specifically trained ones like SEQCODER. To measure performance, the team employed metrics such as accuracy—whether the generated program reproduces the sequence—and precision—how concise the correct program is compared to GZIP compression. The test involved compressing sequences of varying lengths, with synthetic sequences averaging 76 bytes and real sequences capped at 128.
Results showed that even the most powerful models struggled. GPT-4 achieved 69.5% accuracy on high-quality audio but dropped to 36.4% for 8-bit audio and 50.3% for DNA data. LLaMA-3.1-405B performed worse, with accuracies as low as 3.9% for audio and only 24.8% for DNA. In synthetic data, SEQCODER-8B reached 92.5% accuracy with a precision score of 0.56, outperforming traditional tools like GZIP. However, its accuracy on real-world data remained near zero. This discrepancy illustrates the difficulty in transferring success from synthetic benchmarks to more varied and noisy real-world sequences, highlighting the limitations of current training regimes and prompting the need for new strategies.
Overall, this research clearly outlines the complexity of compression via code generation. The KT benchmark provides a rigorous and diverse model reasoning and structure recognition test, exposing the stark divide between synthetic learning environments and real-world applications. The introduced methodology and test set a high bar for future models aiming to unify reasoning with compression, but significant innovation is still required to meet this challenge.
Check out the Paper. All credit for this research goes to the researchers of this project. Also, feel free to follow us on Twitter and don’t forget to join our 85k+ ML SubReddit.
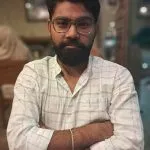
Nikhil is an intern consultant at Marktechpost. He is pursuing an integrated dual degree in Materials at the Indian Institute of Technology, Kharagpur. Nikhil is an AI/ML enthusiast who is always researching applications in fields like biomaterials and biomedical science. With a strong background in Material Science, he is exploring new advancements and creating opportunities to contribute.